What are Emerging Topics in Digitally-driven business models?
- SIX
- Oct 19, 2022
- 5 min read
Updated: Nov 7, 2022
The most general-purpose technologies of our era are digital by nature. On-going technological advancement is probably the most prominent force shaping the modern business landscape. In this blog, we use scholarly literature to explore what are emerging topics in digital-driven business models. We employed bibliometric methods to retrieve and analyse the data accumulated from the Web of Science (WOS). For details of this literature search, please see the working paper by Heshmatisafa & Seppänen (SSRN 2022). Bibliometric approach provides a bird's-eye view of the complicated issues and the stakes on all sides, insights not often shared among practitioners.
Each decade, we confront novel technological advancements. Figure 1 demonstrates the top twenty emerging technologies cited over the past two decades. Here we categorise them as emerging technologies since they are relatively new ones and encompass a great level of uncertainty and novel features.
For instance, business literature has discussed the application of AI since 2006; but only after 2018 the discussion achieved noteworthy growth, both in the number of articles and depth of knowledge. This surge of attention is mainly due to the increased adaptation of AI in novel application domains.
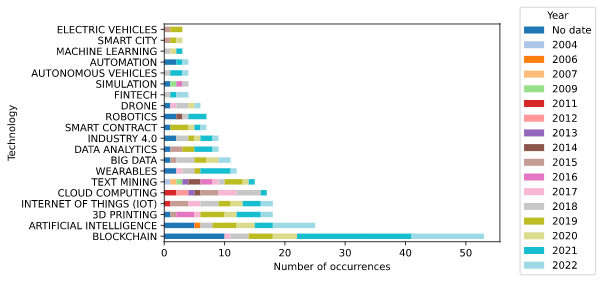
Empirical studies show that technological frontiers have consistently exploited emerging technologies to rapidly respond to market needs and change their value proposition. Emerging technologies are transforming businesses and materialising different components of the value chain. They are shifting economic paradigms and introducing the digital economy into various industries.
Figure 2 below illustrates how the emphasis on emerging technologies and strategies has changed over the past seven years. Technologies such as big data, artificial intelligence, blockchain, industry 4.0, and Internet of Things have been proliferating in the past seven years. These techs are studied alongside dynamic capabilities, value creation, and different business models, which implies that emerging technologies are reshaping business strategies.
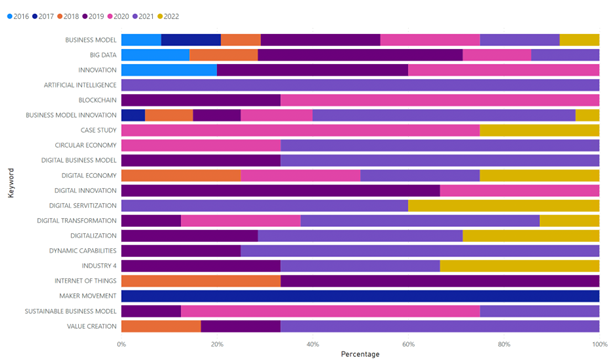
For instance, it is evident that Industrial Internet of Things is facilitating the formation of sustainable business models. This trend can be seen in both Figure 2 and Figure 3. The growing appearance of both circular economy and digital servitization suggests a strategic approach in which business models not only portray the development of new solutions and profits but also address the use of technological advancements for external concerns such as regulation and environmental issues.
Such multiple usages of resources assist firms’ journey to reflect on environmental and economic aspects, stay relevant in future markets and gain public approval. However, to fully utilise the potential of emerging technologies, innovation is essential.
A recent survey by McKinsey indicates that only 4% have already made fundamental changes to their business models; 21% plan on embedding technologies into their current models, while an astounding 64% want to build entirely new digital businesses. This means we may expect to see a considerable shift among industries as they build digital practices from the ground up rather than improving existing models.
The coupling network (Figure 3) demonstrates that innovation is the focal point in almost all clusters. The diagram displays how business model innovation is typically linked to digital transformation and emerging technologies. Aside from typical characteristics of emerging technologies (i.e., creating a digital economy, developing novel solutions, multi-purpose, and stimulating business model innovation), they are heavily nested. Figure 3 also demonstrates, that some techs are directly or indirectly connected to one another.
These converging technologies gave birth to electric vehicles and blurred lines between disparate technologies. Electric vehicles run on alternative fuels; they tap into an interconnected electricity grid while interacting with IoT techs to analyse and transmit the data. Such complex system of systems comes with notable drawbacks, and we can see that scholars rightfully discourse challenges more than opportunities.
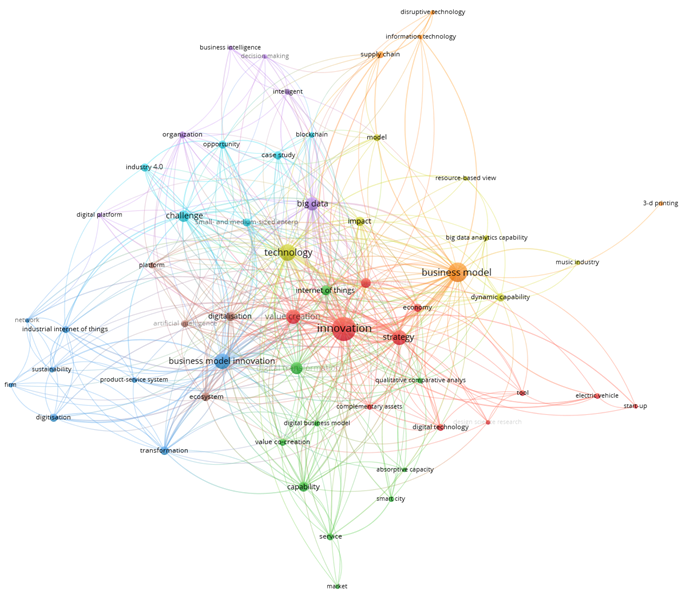
In short, rapid digital innovation enabled by emerging technologies alters current value propositions and enables firms to develop innovative solutions to address various business and market needs. However, every emerging technology is at a different maturity level and level of uncertainty, and it is unlikely that they can deliver significant business value independent from one another.
Nonetheless, businesses are aggressively using emerging technologies and plan to be “fast followers”. Thus, we may propose that companies consider researching emerging technologies and their potential impacts on business goals and objectives.
We also suggest that early adaptors could rethink their strategy and redesign their business models while taking into consideration at least three subjects: technology system maturity, development and integration time, and human interaction, all three are discussed below in more detail.
Technology system maturity
The maturity level of a specific technology most likely depends on the maturity of complementary technologies. Companies must investigate the technological dependency of proposed solutions from the resource-based view. For example, autonomous cars (depending on the level of automation) rely on other technological development such as cloud computing, sensors, machine learning systems, a trip mapping system, machine vision, machine learning and many more.
For instance, self-driving cars constantly feed data to advanced neural network algorithms to increase precision and derive meaning in real-time. However, current network techs are not sufficient in crowded areas. All this implies high-level readiness issues in the overall technological system and its sub-systems.
Development and integration
Generic AI systems add little value to any business; thus, it requires tailoring and configuration to be able to address a company’s needs and specific knowledge domain. If knowledge domain has not been modelled, it will typically require many months to architect. Even once the systems have been built, there may be the issue of integrating AI systems into a firm’s organization. In most cases, fitting AI systems with existing business processes and IT architecture will require significant planning and time for adaptation.
Moreover, the transition from pilots and prototypes to production systems for AI can be difficult and time-consuming. Also, some redesigning business processes and adjusting to human tasks might be needed. For instance, AI is capable of processing individual tasks and not an entire business process, thus necessitating atomised business processes.
Human interaction
Lastly, there are human challenges to consider. Very few technologies are yet fully autonomous. Instead, they are focused on the augmentation of and by human workers. Typically, new roles and skills are required for the humans to work alongside them, and it will usually require upskilling workers on the new process and system.
For example, companies offering "Robo-advice" to their customers have often attempted to get human advisors to shift their focus from standard counsels to more specialised decisions and actions. In the case of full automation, the system needs to observe and learn the interactions between the system and human users. During this, known as interaction learning, new datasets are often fed to the algorithms, which can take months or years to train.
Concluding remarks
Our new digital world compels digital transformation, and the use of emerging technology is almost inevitable. Firms must constantly decide what technology to keep, what to scale and what to sunset. Some technologies require further development, while others are quite mature and have been available in some form for many years. Holding back from technologies to mature enough and become widely available puts firms behind the curve.
Emerging technologies come with their benefits and challenges. They perform better with complementary technologies. When complementary technologies are realised, they require significant planning and time for development and integration.
Consequently, by the time a late adopter has done all the necessary preparation, earlier adopters have already gained considerable market share and can operate at lower costs with better performance. Hence, firms need to keep up with current IT trends and informed by their complexity to make the right decision at the right time.
Saeid Heshmatisafa & Marko Seppänen
Unit of Information and Knowledge Management
Tampere University
September 26, 2022
Comments